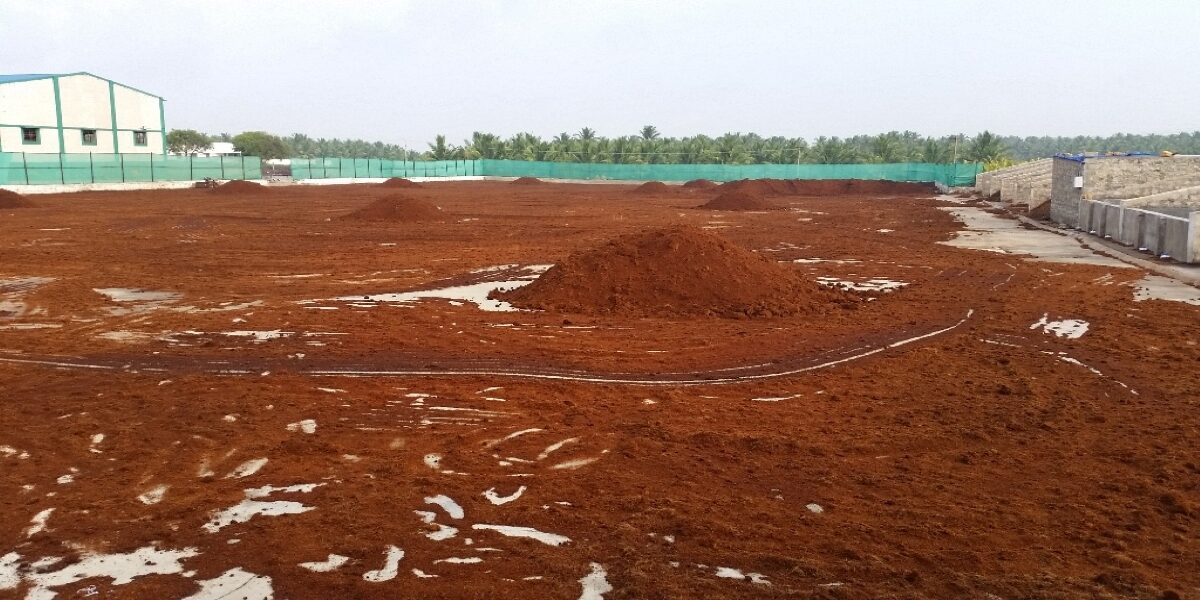
Analyzing data that has not been carefully screened for such problems can produce misleading results. Thus, the representation and quality of data is first and foremost before running any analysis. Often, data preprocessing is the most important phase of a machine learning project, especially in computational biology.
If there is much irrelevant and redundant information present or noisy and unreliable data, then knowledge discovery during the training phase is more difficult. Data preparation and filtering steps can take considerable amount of processing time. Examples of data preprocessing include cleaning, instance selection, normalization, one hot encoding, transformation, feature extraction and selection, etc
Data preprocessing may affect the way in which outcomes of the final data processing can be interpreted. This aspect should be carefully considered when interpretation of the results is a key point, such in the multivariate processing of chemical data.
- Client : Boss Coirs
- Live Demo : https://bosscoirs.com/
- Category : Boss
- Date : December 20, 2017